Julian Dierkes
Chair for AI Methodology, RWTH Aachen University.
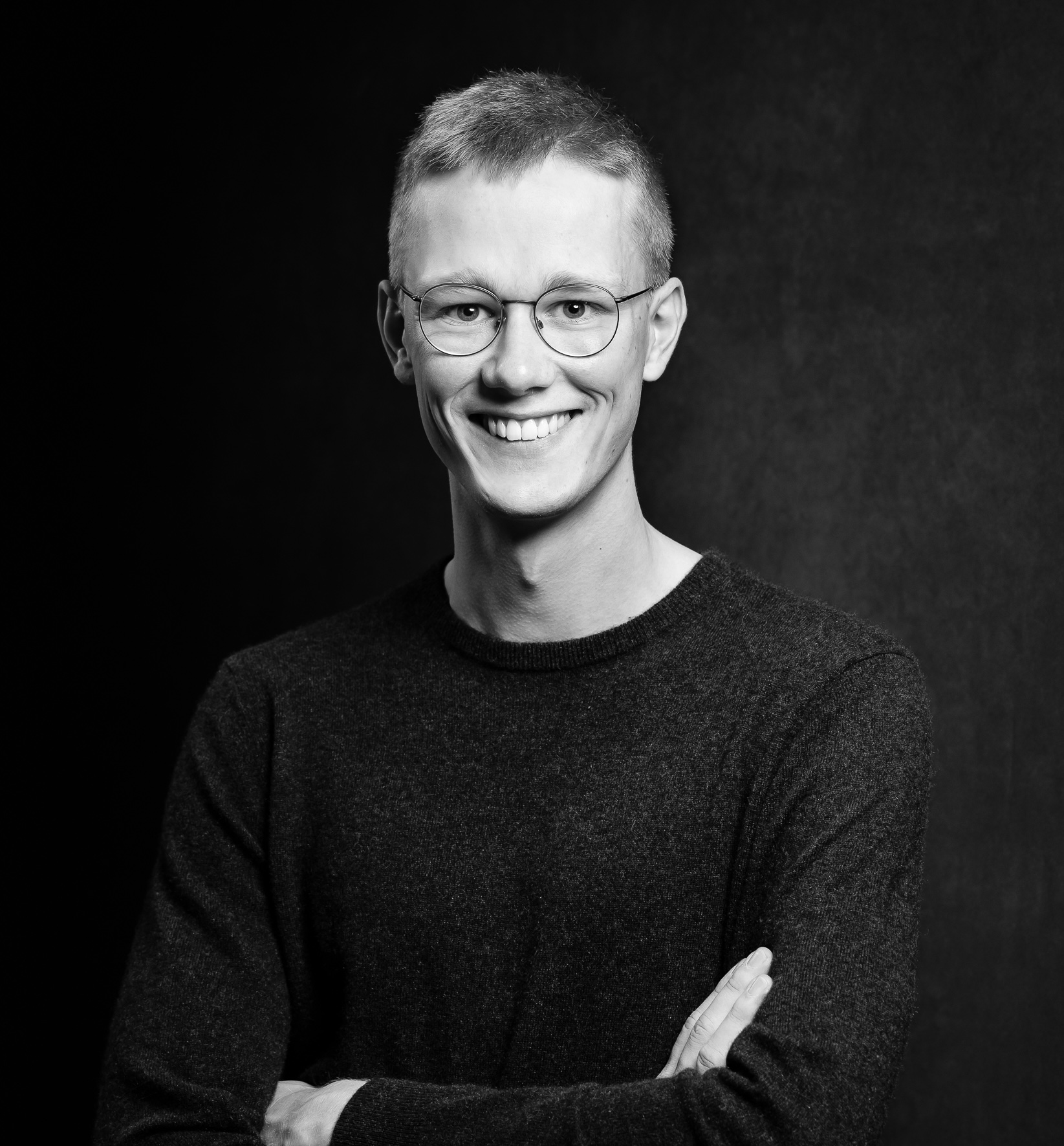
I’m a PhD student at RWTH Aachen University, working on Automated Machine Learning for Reinforcement Learning under the supervision of Holger Hoos. My research focuses on increasing the practical applicability of Reinforcement Learning by automating the many design decisions required to learn effective policies.
With my research I want to bridge foundational research in Reinforcement Learning with the practical advances already developed in the Automated Machine Learning community. Ultimately, I hope this work will transform Reinforcement Learning into more of an engineering discipline, moving away from its reputation as a ‘black box.’
Before my PhD, I worked on fine-tuning large speech recognition systems for low-resource languages. I’m very curious about applying Reinforcement Learning to large foundational models as well to enhance their capabilities beyond text and speech.
Besides my research, I enjoy exploring some of the more philosophical questions related to the development of intelligence. I’m particularly fascinated by how biological brains evolved to be so powerful, and how we’re attempting to replicate their abilities through artificial, mostly very different, methods.
news
May 01, 2025 | I have been awarded a nine-month scholarship by the German Academic Exchange Service (DAAD) and will be joining MILA in Montreal as an incoming student starting in September. I will be working in Professor Castro’s lab on the dependencies between RL hyperparameters and environment properties. Super excited to go! |
---|---|
Jan 01, 2025 | I am now part of the AI Grid initiative, which aims to strengthen the exchange and synergies between AI Doctorates in Germany. |
Oct 01, 2024 | Our joint work about the Automated RL Benchmark was accepted at the European Workshop of Reinforcement Learning this year and is now available at Arxiv. The benchmark contains lightning fast implementations of three common RL algorithms in JAX, build specifically to benchmark AutoRL methods in a consistent setting. |
Jun 22, 2024 | I am co-organising the AutoRL workshop at ICML this year with an amazing team. Very much looking forward to the workshop and to meet all the people excited about AutoRL. |
Mar 30, 2024 | My first AutoRL paper as a PhD student was accepted at this year’s Reinforcement Learning Conference, where we explored the potential of jointly optimising hyperparameters and reward functions. I am very excited to present our work this August in Amherst! |
selected publications
- EWRLARLBench: Flexible and Efficient Benchmarking for Hyperparameter Optimization in Reinforcement Learning17th European Workshop on Reinforcement Learning. GitHub Repo can be found here , Oct 2024
- Combining Automated Optimisation of Hyperparameters and Reward ShapeProc. of the Reinforcement Learning Journal. GitHub Repo can be found here , Aug 2024